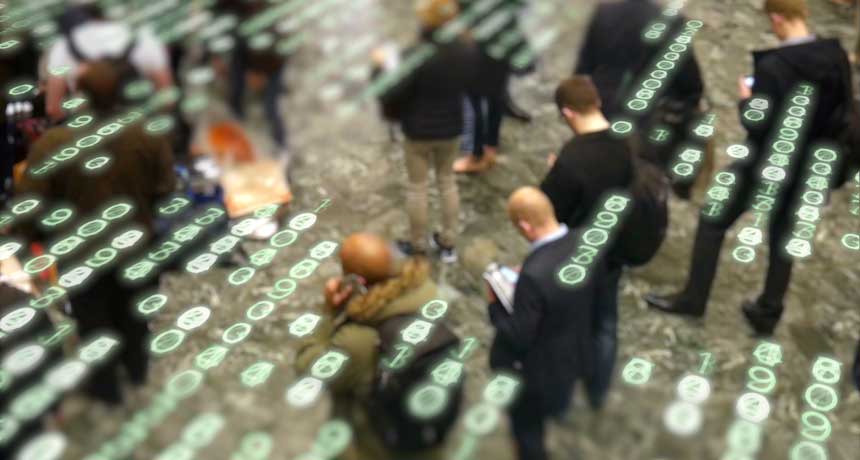
As per a recent survey in 2021, 76% of enterprises have preferred and prioritized AI and ML integration projects over any other IT initiatives, thus making ML as one of the popular engines churning the business. As fancy as Machine learning sounds, there’s still a vast scope in understanding its power to harness the information coming from consumers across businesses.
In layman’s terms, businesses can leverage analytics to scale by embedding ML algorithms in various process workflows to transform their operations in a faster, predictive, innovative and customer-friendly way. But is it all? No, one needs deep expertise and core competencies to be able to utilize ML in the best way possible for business. A big misconception that comes with harnessing ML is that it runs on the same algorithm for across all niche of business. This is so untrue. ML-driven technology used in Netflix can be so different than what ML-algorithm may feed in the debt collection industry.
Unlike Netflix and Amazon who are vested much in using ML to fuel up their recommendation engines using the historic customer data, movie characteristics as actor, actress, director, producer, story genres, co stars etc to be effective. These algorithms have been in product for more than 10+ years and move of us have forgotten the poor recommendation these produced in the initial years. Similarly one has to be ready to invest time and money to create lot of meaningful debt collection variables from traditional, alternative data to understand the consumer behavior. As the challenge faced by Netflix and Amazon this effort requires significant resources and kkilled team to harvest the data followed by harvesting the ML engine recommendation and implementing in a very practical manner to see any meaningful results.
There are a lot of pros with leveraging ML-driven collection strategy in the industry but with it, also comes few cons if not integrated or adapted well. The Auto ML features may backfire and steer more calls if not configured properly resulting in call regression, more drop-offs and incorrect forecasting of debt recovery. Also tunning a ML with new unproven or new digital channel content and digital frequency recommendation would be extremely dangerous too. This makes us think of how assertive it is to have the right set of data scientists, analysts and developers with extensive programming knowledge to build ML models with high-efficiency and super-productive sustainability.
Now that’s one prerequisite to adapting ML but having a right mindset is equally imperative to define a precise requirement of your business before adapting ML-driven models to your debt collection strategy. Every industry’s requirement is different and every ML-predictive model set is different. One can never categorize ML under one broad spectrum of technology. Machine learning in debt collection process usually identifies patterns and anomalies in the data from multiple communication channels in order to suggest the best mode of communication for each consumer and the optimal time to reach out to them thus causing less friction in the debt recovery calls. Prior to ML, the strategy wasn’t this smooth, there were scheduled calls irrespective of customer’s preference and a lot of follow-ups sometimes just to reach the right point of contact. A model to predict consumer behavior and preference was missing which now has been added owing to ML-driven model in the collection industry. The major challenge earlier without ML was consumers not picking up the call and receiving warning letters owing to which a number of agencies flouted the law and were blacklisted due to complaints and compliance risk. Machine learning has widely mitigated this risk and reduced the cost of operations owing to less drop-offs and better consumer experience.
Consumer preferences have changed over time. He, now, prefers a wide range of communication channel and a single-click seamless platform for making payments. ML can evaluate consumer’s speech in real time to enable agent make informed decisions. Not only this, the algorithm identifies the account with highest pay propensity and prioritizes it first for quick debt recovery with minimal cost involved. But what supplements this level of technology-driven prediction?
That’s where having a significant team that can feed back relevant and systematic data into the system to get best results is critical. Not only this, having an expert team to be capable to use this analytical data in real-time is equally important. The feeding of historical data to generate such-level of analysis doesn’t happen in weeks or even months?
It is a regressive process that can take even up to an year or more. The evaluation and validation of historical data needs a panel of expert data scientists and analysts who would learn, optimize and streamline the feedback to move to the next generation collection strategy. The expert engineering and attribution of analytical data along with production deployment and evaluation takes time, money and patience to yield the desired results or revenue to the business. This is where most companies lose faith. Having a team of robust data scientists isn’t just enough but keeping them motivated and guiding them through this span of months or year is essential especially when you are not seeing successful results immediately. Making sure the team stays away from the ‘shiny object syndrome’ is important before jumping onto conclusions or finding a next interesting project or strategy to generate revenue.
Have such a team that continuously churns customer insight data to feed the algorithm and build right data sets to be able to leverage ML-model successfully in the business growth. A better and assured way to this is to buy some help from outside and gradually incorporate it in your system while building a unique platform for your business. There have been examples in the past when legacy companies have thought of moving to cloud and created their own MLOps with a ton of money and manpower invested, resulting in crash while dealing with such sensitive data in lesser time and restricted expertise. To prevent ourselves from incurring this, it is usually advisable to take expert help and have vendors integrate your operations and workflows end-to end as they come with that bandwidth and expertise with little or no room for failure.
With buying such a ML-driven model product and gradually building inhouse platform is a much durable way of keeping the business afloat while having it move to cloud and run digitally. Applying the practices of a third-party vendor model increases the simplicity of the management process and automates the deployment of ML models in production environment in a much hassle-free and cost as well as time-effective way. A business model can anytime adapt their own framework in sync to the existing ML model and pilot it successfully with the help of expert panelists in the AI and ML-driven base technology.
Watch out for the next article on the Collection AI Series!!!
About Abhishek Goel
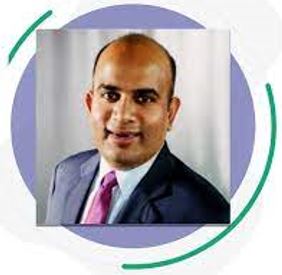
Abhishek is the CEO and Founder and Consumer Behavior Expert for Dasceq. At Dasceq, he is on a mission to contribute big to the Digital Debt Collections underpinned by ‘Empathy-driven Digital Collections’ approach. Abhishek has over 16 years of experience in developing and implementing AI/ML innovative solutions delivering ROI for 300+ optimization projects, $3+ Billions. He is passionate teach data science and is an adjunct professor and advisor, at graduate programs at Springboard.com, SMU, Dallas and UTD Dallas.
Facebook Comments